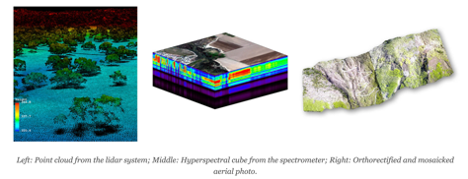
By Luke Browne
This summer, a group of YSE PhD students, postdocs, and a recent Yale College graduate competed in an international competition aimed at developing the best machine learning algorithms for identifying tree species using remote sensing images. The team, known as Más JALApeñoS, came in first place (link is external) for classifying tree crowns from forest data originating in Alabama, Florida, and Virginia based on the cross-entropy evaluation metric, which estimates how well-calibrated the model predictions are to the truth.
The IdTrees competition (link is external) first began in 2017 and is now on its second iteration, with the best models from the first run of the competition achieving upwards of 92% accuracy in species identification. With the skyrocketing availability of remote sensing imaging from drones and satellites, translating this data into information that can be used to better understand and manage forests is a major challenge. Achieving reliable species identification with remote sensing images would unlock huge potential for biodiversity surveys and monitoring at scales that were previously not feasible. The team included YSE PhD students Megan Sullivan, Akshay Surendra, Alex Polussa from the Comita and Bradford labs, YSE postdoctoral researchers Sergio Estrada & Luke Browne from the Comita lab, and recent Yale College graduate Jasmine Liu.